

These methods are based on the learning of a great quantity of training samples of known fault types to realise the classification and diagnosis of unknown fault data samples. At present, an enormous amount of research mostly adopts the fault classification and recognition method based on supervised learning, such as artificial neural network (ANN), SVM, fault tree analysis (FTA), deep learning (DL), random forest (RF) etc. In addition, there are many intelligent algorithms for fault classification and recognition. It has good denoising effect and strong ability to extract key feature information from vibration signal. In essence, it is used to construct and solve variational problems, to replace the recursive decomposition mode, which has greatly improved the mode mixing problem and the robustness to noise. proposed a novel signal decomposition method, VMD. It leads to serious mode mixing and end effect, which reduces the diagnostic accuracy of a pattern recognition process. In this process, the error of envelope estimation can be accumulated. However, EMD and LMD algorithms belong to the recursive decomposition mode in principle. proposed the LMD algorithm to process fault signals and to extract fault features, and finally used the kernel fuzzy C-means (KFCM) method to diagnose gear and broken teeth, and achieved a good classification effect. The EMD algorithm has been proposed to extract fault features in reference and the support vector machine (SVM) algorithm has been used to classify bearing and gear faults, achieving high accuracy. At present, signal decomposition algorithms such as empirical mode decomposition (EMD), local mean decomposition (LMD) and variable mode decomposition (VMD) are widely used in fault signal processing and fault feature extraction.

In fact, using the vibration signal for fault diagnosis is the pattern recognition of fault information, which mainly consists of two parts: fault feature information extraction and identification.
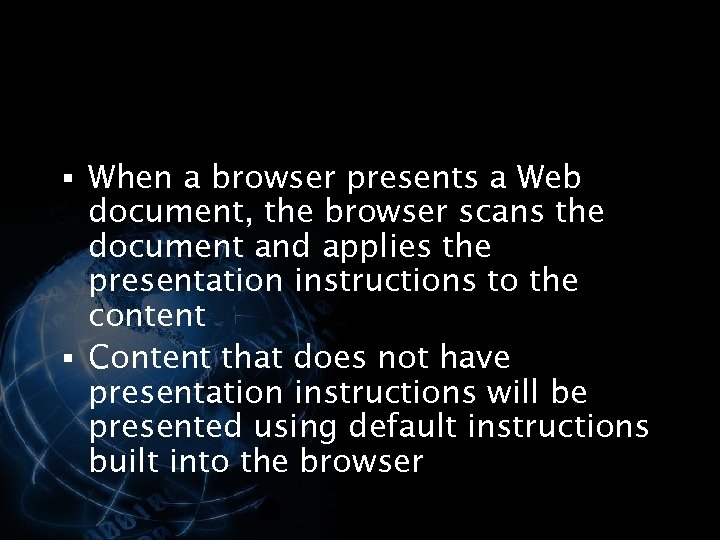
Therefore, processing the original vibration signal, separating and extracting key feature information and improving the correct rate of fault pattern recognition are the keys to current fault diagnosis research. When a fault occurs, the vibration signal is usually superimposed non-linearly between different excitation signals and contains significant noise, which makes it difficult to capture and extract the weak fault-sensitive features. Due to the long-term high load, high speed, heavy impact and loud noise, various faults will inevitably occur. Rolling bearings are widely used in rotating machinery. The accuracy of fault classification was improved obviously. Finally, different fault classification methods are compared, and the conclusions drawn from the experiment show that the method can achieve good results in bearing fault diagnosis. The clustering centre of each fault type under the optimal classification result was obtained, and the fault diagnosis model was established. The PSO algorithm was used to solve the clustering model. Then, the kernel function parameters and the initial clustering centre were used as optimisation variables. The singular value decomposition method was used to extract a characteristic value corresponding to the main fault types of bearings from the optimal decomposition results, and faulty feature sample space was established. Firstly, by calculating the centre frequency and Pearson correlation coefficient of each mode function of VMD, the decomposition level K of VMD is determined, and the optimal decomposition result is obtained. In order to make accurate judgements of rolling bearing main fault types using the small sample size fault data set, a novel approach is put forward that combines particle swarm optimisation kernel fuzzy C-means (PSO-KFCM) and variational mode decomposition (VMD). IET Generation, Transmission & Distribution.IET Electrical Systems in Transportation.IET Cyber-Physical Systems: Theory & Applications.IET Collaborative Intelligent Manufacturing.CAAI Transactions on Intelligence Technology.
